Choosing the Best GPU for Deep Learning in 2020
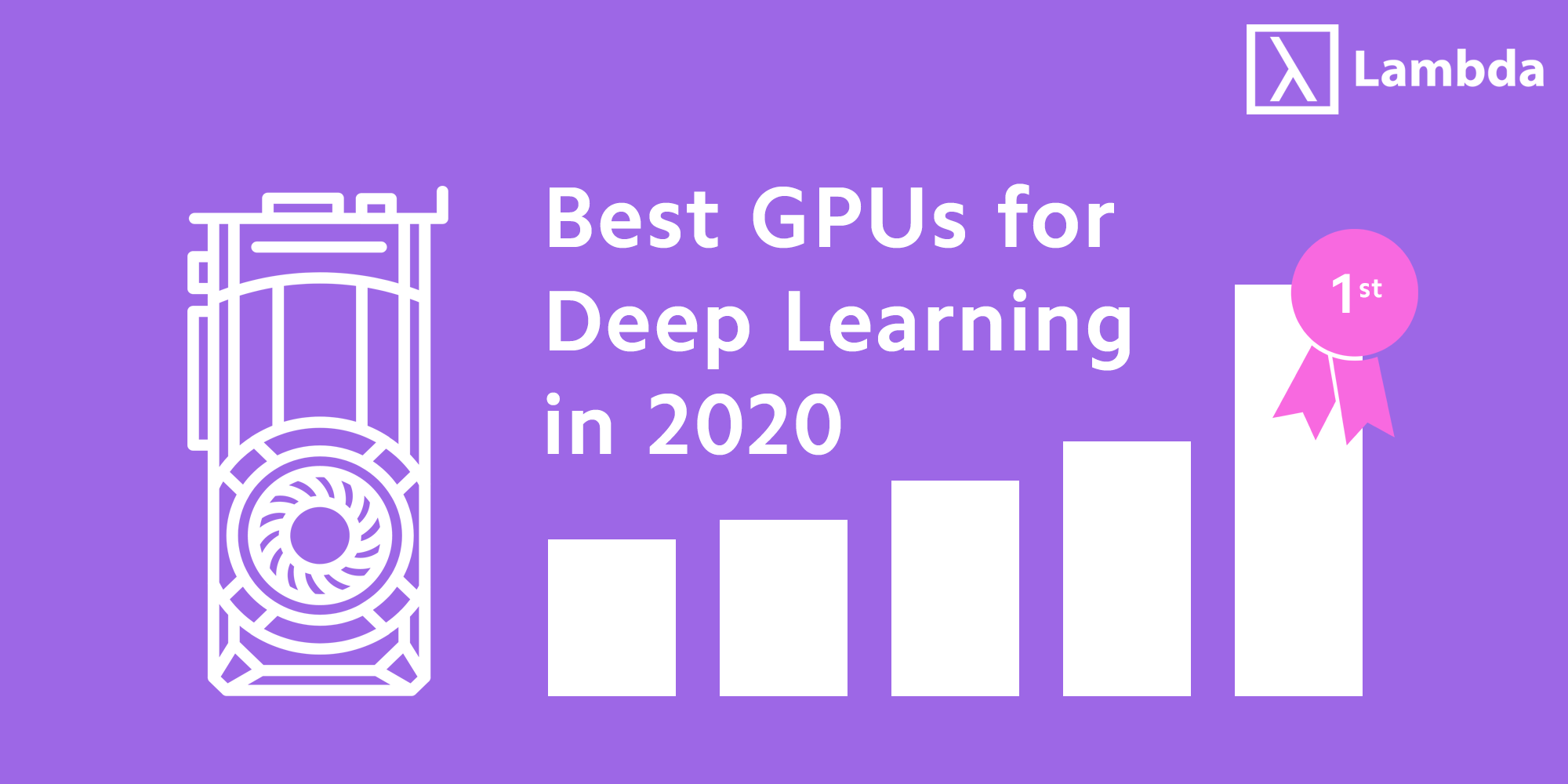
State-of-the-art (SOTA) deep learning models have massive memory footprints. Many GPUs don't have enough VRAM to train them. In this post, we determine which GPUs can train state-of-the-art networks without throwing memory errors. We also benchmark each GPU's training performance.
TLDR
The following GPUs can train all SOTA language and image models as of February 2020:
- RTX 8000: 48 GB VRAM, ~$5,500.
- RTX 6000: 24 GB VRAM, ~$4,000.
- Titan RTX: 24 GB VRAM, ~$2,500.
The following GPUs can train most (but not all) SOTA models:
- RTX 2080 Ti: 11 GB VRAM, ~$1,150. *
- GTX 1080 Ti: 11 GB VRAM, ~$800 refurbished. *
- RTX 2080: 8 GB VRAM, ~$720. *
- RTX 2070: 8 GB VRAM, ~$500. *
The following GPU is not a good fit for training SOTA models:
- RTX 2060: 6 GB VRAM, ~$359.
* Training on these GPUs requires small batch sizes, so expect lower model accuracy because the approximation of a model's energy landscape will be compromised.
Image models
Maximum batch size before running out of memory
Model / GPU | 2060 | 2070 | 2080 | 1080 Ti | 2080 Ti | Titan RTX | RTX 6000 | RTX 8000 |
---|---|---|---|---|---|---|---|---|
NasNet Large | 4 | 8 | 8 | 8 | 8 | 32 | 32 | 64 |
DeepLabv3 | 2 | 2 | 2 | 4 | 4 | 8 | 8 | 16 |
Yolo v3 | 2 | 4 | 4 | 4 | 4 | 8 | 8 | 16 |
Pix2Pix HD | 0* | 0* | 0* | 0* | 0* | 1 | 1 | 2 |
StyleGAN | 1 | 1 | 1 | 4 | 4 | 8 | 8 | 16 |
MaskRCNN | 1 | 2 | 2 | 2 | 2 | 8 | 8 | 16 |
*The GPU does not have enough memory to run the model. |
Performance, measured in images processed per second
Model / GPU | 2060 | 2070 | 2080 | 1080 Ti | 2080 Ti | Titan RTX | RTX 6000 | RTX 8000 |
---|---|---|---|---|---|---|---|---|
NasNet Large | 7.3 | 9.2 | 10.9 | 10.1 | 12.9 | 16.3 | 13.9 | 15.6 |
DeepLabv3 | 4.4 | 4.82 | 5.8 | 5.43 | 7.6 | 9.01 | 8.02 | 9.12 |
Yolo v3 | 7.8 | 9.15 | 11.08 | 11.03 | 14.12 | 14.22 | 12.8 | 14.22 |
Pix2Pix HD | 0.0* | 0.0* | 0.0* | 0.0* | 0.0* | 0.73 | 0.71 | 0.71 |
StyleGAN | 1.92 | 2.25 | 2.6 | 2.97 | 4.22 | 4.94 | 4.25 | 4.96 |
MaskRCNN | 2.85 | 3.33 | 4.36 | 4.42 | 5.22 | 6.3 | 5.54 | 5.84 |
*The GPU does not have enough memory to run the model. |
Language models
Maximum batch size before running out of memory
Model / GPU | Units | 2060 | 2070 | 2080 | 1080 Ti | 2080 Ti | Titan RTX | RTX 6000 | RTX 8000 |
---|---|---|---|---|---|---|---|---|---|
Transformer Big | Tokens | 0* | 2000 | 2000 | 4000 | 4000 | 8000 | 8000 | 16000 |
Conv. Seq2Seq | Tokens | 0* | 2000 | 2000 | 3584 | 3584 | 8000 | 8000 | 16000 |
unsupMT | Tokens | 0* | 500 | 500 | 1000 | 1000 | 4000 | 4000 | 8000 |
BERT Base | Sequences | 8 | 16 | 16 | 32 | 32 | 64 | 64 | 128 |
BERT Finetune | Sequences | 1 | 6 | 6 | 6 | 6 | 24 | 24 | 48 |
MT-DNN | Sequences | 0* | 1 | 1 | 2 | 2 | 4 | 4 | 8 |
*The GPU does not have enough memory to run the model. |
Performance
Model / GPU | Units | 2060 | 2070 | 2080 | 1080 Ti | 2080 Ti | Titan RTX | RTX 6000 | RTX 8000 |
---|---|---|---|---|---|---|---|---|---|
Transformer Big | Words/sec | 0* | 4597 | 6317 | 6207 | 7780 | 8498 | 7407 | 7507 |
Conv. Seq2Seq | Words/sec | 0* | 7721 | 9950 | 5870 | 15671 | 21180 | 20500 | 22450 |
unsupMT | Words/sec | 0* | 1010 | 1212 | 1824 | 2025 | 3850 | 3725 | 3735 |
BERT Base | Ex./sec | 34 | 47 | 58 | 60 | 83 | 102 | 98 | 94 |
BERT Finetue | Ex./sec | 7 | 15 | 18 | 17 | 22 | 30 | 29 | 27 |
MT-DNN | Ex./sec | 0* | 3 | 4 | 8 | 9 | 18 | 18 | 28 |
*The GPU does not have enough memory to run the model. |
Results normalized by Quadro RTX 8000
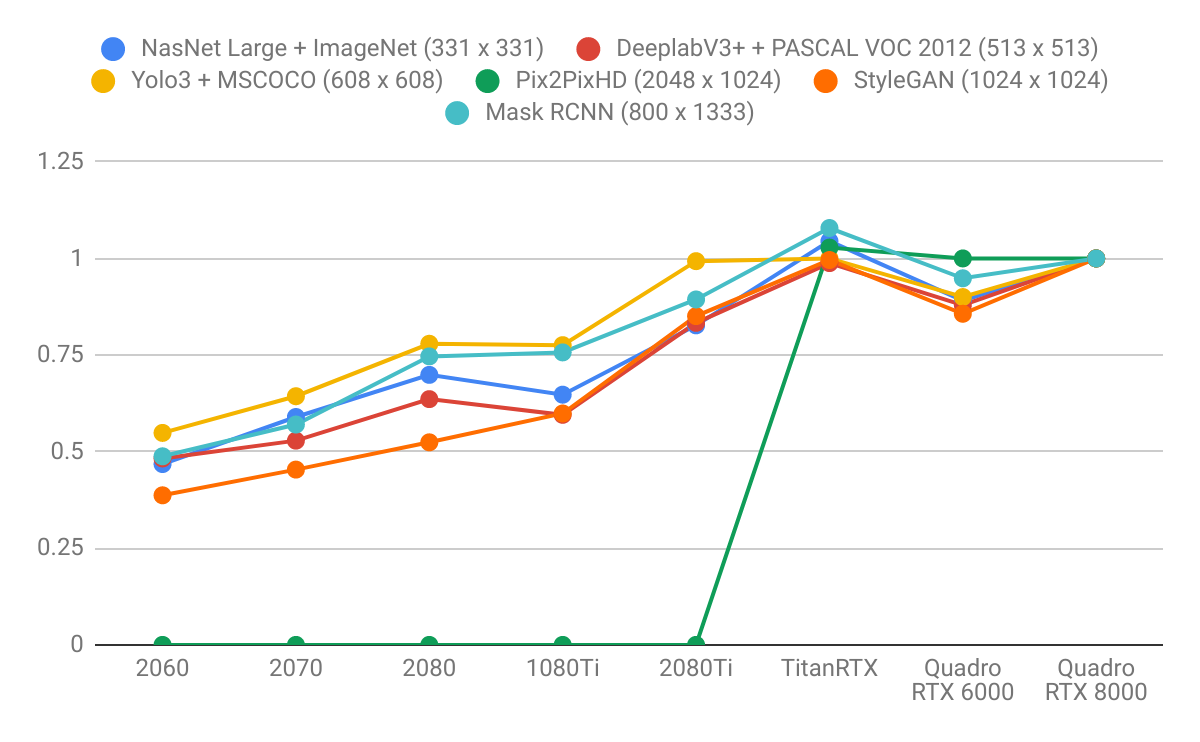
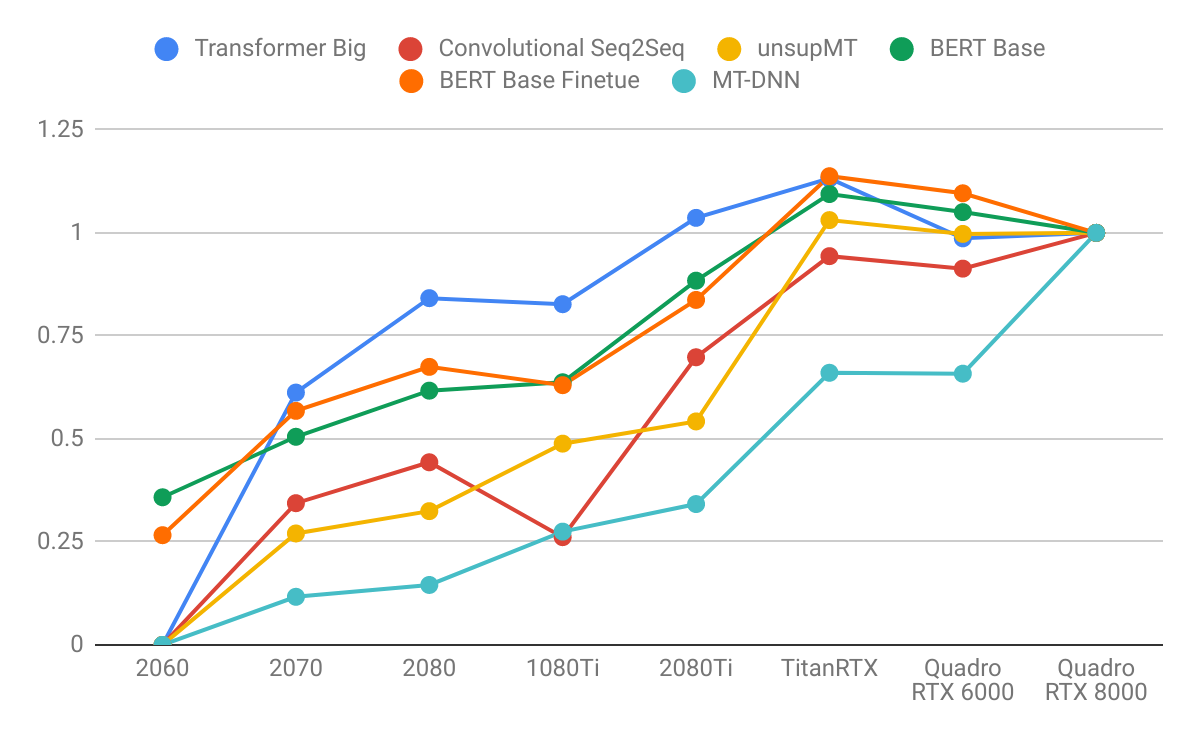
Conclusions
- Language models benefit more from larger GPU memory than image models. Note how the right diagram is steeper than the left. This indicates that language models are more memory-bound and image models are more computationally bounded.
- GPUs with higher VRAM have better performance because using larger batch sizes helps saturate the CUDA cores.
- GPUs with higher VRAM enable proportionally larger batch sizes. Back-of-the-envelope calculations yield reasonable results: GPUs with 24 GB of VRAM can fit a ~3x larger batches than a GPUs with 8 GB of VRAM.
- Language models are disproportionately memory intensive for long sequences because attention is quadratic to the sequence length.
GPU Recommendations
- RTX 2060 (6 GB): if you want to explore deep learning in your spare time.
- RTX 2070 or 2080 (8 GB): if you are serious about deep learning, but your GPU budget is $600-800. Eight GB of VRAM can fit the majority of models.
- RTX 2080 Ti (11 GB): if you are serious about deep learning and your GPU budget is ~$1,200. The RTX 2080 Ti is ~40% faster than the RTX 2080.
- Titan RTX and Quadro RTX 6000 (24 GB): if you are working on SOTA models extensively, but don't have budget for the future-proofing available with the RTX 8000.
- Quadro RTX 8000 (48 GB): you are investing in the future and might even be lucky enough to research SOTA deep learning in 2020.
Lambda offers GPU laptops and workstations with GPU configurations ranging from a single RTX 2070 up to 4 Quadro RTX 8000s. Additionally, we offer servers supporting up to 10 Quadro RTX 8000s or 16 Tesla V100 GPUs.
Footnotes
Image Models
Model | Task | Dataset | Image Size | Repo |
---|---|---|---|---|
NasNet Large | Image Classification | ImageNet | 331x331 | Github |
DeepLabv3 | Image Segmentation | PASCAL VOC | 513x513 | GitHub |
Yolo v3 | Object Detection | MSCOCO | 608x608 | GitHub |
Pix2Pix HD | Image Stylization | CityScape | 2048x1024 | GitHub |
StyleGAN | Image Generation | FFHQ | 1024x1024 | GitHub |
MaskRCNN | Instance Segmentation | MSCOCO | 800x1333 | GitHub |
Language Models
Model | Task | Dataset | Repo |
---|---|---|---|
Transformer Big | Supervised machine translation | WMT16_en_de | GitHub |
Conv. Seq2Seq | Supervised machine translation | WMT14_en_de | GitHub |
unsupMT | Unsupervised machine translation | NewsCrawl | GitHub |
BERT Base | Language modeling | enwik8 | GitHub |
BERT Finetune | Question and answer | SQUAD 1.1 | GitHub |
MT-DNN | GLUE | GLUE | GitHub |