Train & fine-tune models on a GPU cloud built for AI workloads
NVIDIA GPUs
Featuring access to NVIDIA H100, A100 & A10 Tensor Core GPUs. Additional instance types include NVIDIA RTX A6000, RTX 6000 & NVIDIA V100 Tensor Core GPU.
Multi-GPU Instances
Train and fine-tune AI models across instance types that make sense for your workload & budget: 1x, 2x, 4x & 8x NVIDIA GPU instances available.
Lambda Cloud API
Launch, terminate and restart instances from an easy-to-use developer-friendly API. Find out more in our API developer docs
GPU instances pre-configured for machine learning
One-click Jupyter access
Quickly connect to NVIDIA GPU instances directly from your browser.
Pre-installed with popular ML frameworks
Ubuntu, TensorFlow, PyTorch, NVIDIA CUDA, and NVIDIA cuDNN come ready to use with Lambda Stack
On-demand GPU cloud pricing*
Access high-power GPUs when you need them. Pay by the minute with no egress fees.
GPUs | VRAM/GPU | vCPUs | RAM | STORAGE | PRICE/GPU/HR* |
On-demand 1x NVIDIA GH200 | 96 GB | 64 | 432 GiB | 4 TiB SSD | $3.19 / GPU / hr |
On-demand 8x NVIDIA H100 SXM | 80 GB | 208 | 1800 GiB | 22 TiB SSD | $2.99 / GPU / hr |
Reserved 8x NVIDIA H100 SXM | 80 GB | 208 | 1800 GiB | 22 TiB SSD | CONTACT SALES |
On-demand 4x NVIDIA H100 SXM | 80 GB | 104 | 900 GiB | 11 TiB SSD | $3.09 / GPU / hr |
On-demand 2x NVIDIA H100 SXM | 80 GB | 52 | 450 GiB | 5.5 TiB SSD | $3.19 / GPU / hr |
On-demand 1x NVIDIA H100 SXM | 80 GB | 26 | 225 GiB | 2.75 TiB SSD | $3.29 / GPU / hr |
On-demand 1x NVIDIA H100 PCIe | 80 GB | 26 | 225 GiB | 1 TiB SSD | $2.49 / GPU / hr |
On-demand 8x NVIDIA A100 SXM | 80 GB | 240 | 1800 GiB | 19.5 TiB SSD | $1.79 / GPU / hr |
On-demand 8x NVIDIA A100 SXM | 40 GB | 124 | 1800 GiB | 5.8 TiB SSD | $1.29 / GPU / hr |
On-demand 1x NVIDIA A100 SXM | 40 GB | 30 | 220 GiB | 512 GiB SSD | $1.29 / GPU / hr |
On-demand 4x NVIDIA A100 PCIe | 40 GB | 120 | 900 GiB | 1 TiB SSD | $1.29 / GPU / hr |
On-demand 2x NVIDIA A100 PCIe | 40 GB | 60 | 450 GiB | 1 TiB SSD | $1.29 / GPU / hr |
On-demand 1x NVIDIA A100 PCIe | 40 GB | 30 | 225 GiB | 512 GiB SSD | $1.29 / GPU / hr |
On-demand 1x NVIDIA A10 | 24 GB | 30 | 226 GiB | 1.3 TiB SSD | $0.75 / GPU / hr |
On-demand 4x NVIDIA A6000 | 48 GB | 56 | 400 GiB | 1 TiB SSD | $0.80 / GPU / hr |
On-demand 2x NVIDIA A6000 | 48 GB | 28 | 200 GiB | 1 TiB SSD | $0.80 / GPU / hr |
On-demand 1x NVIDIA A6000 | 48 GB | 14 | 100 GiB | 512 GiB SSD | $0.80 / GPU / hr |
On-demand 8x NVIDIA Tesla V100 | 16 GB | 88 | 448 GiB | 5.8 TiB SSD | $0.55 / GPU / hr |
On-demand 1x NVIDIA Quadro RTX 6000 | 24 GB | 14 | 46 GiB | 512 GiB SSD | $0.50 / GPU / hr |
*plus applicable sales tax
Trusted by thousands of AI developers
ML Engineers & Researchers love Lambda On-Demand Cloud for its simplicity, speed & ML-first user experience.
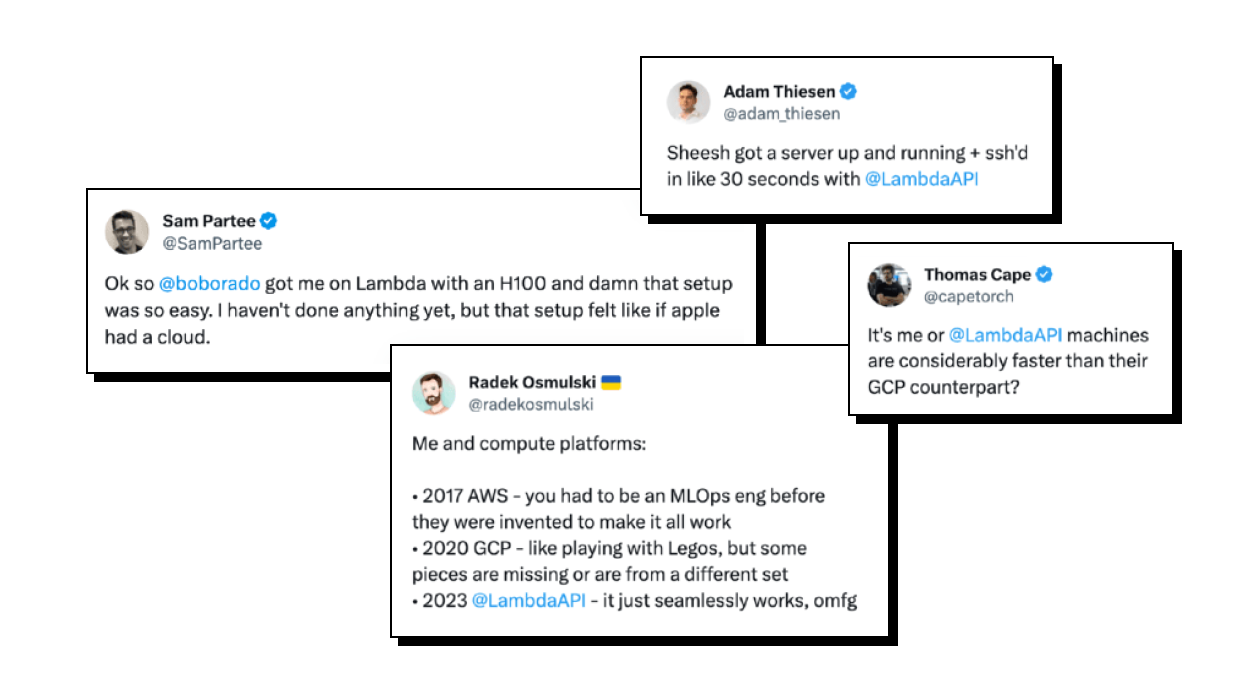
NVIDIA H100s are now available on-demand
Lambda is one of the first cloud providers to make NVIDIA H100 Tensor Core GPUs available on-demand in a public cloud.
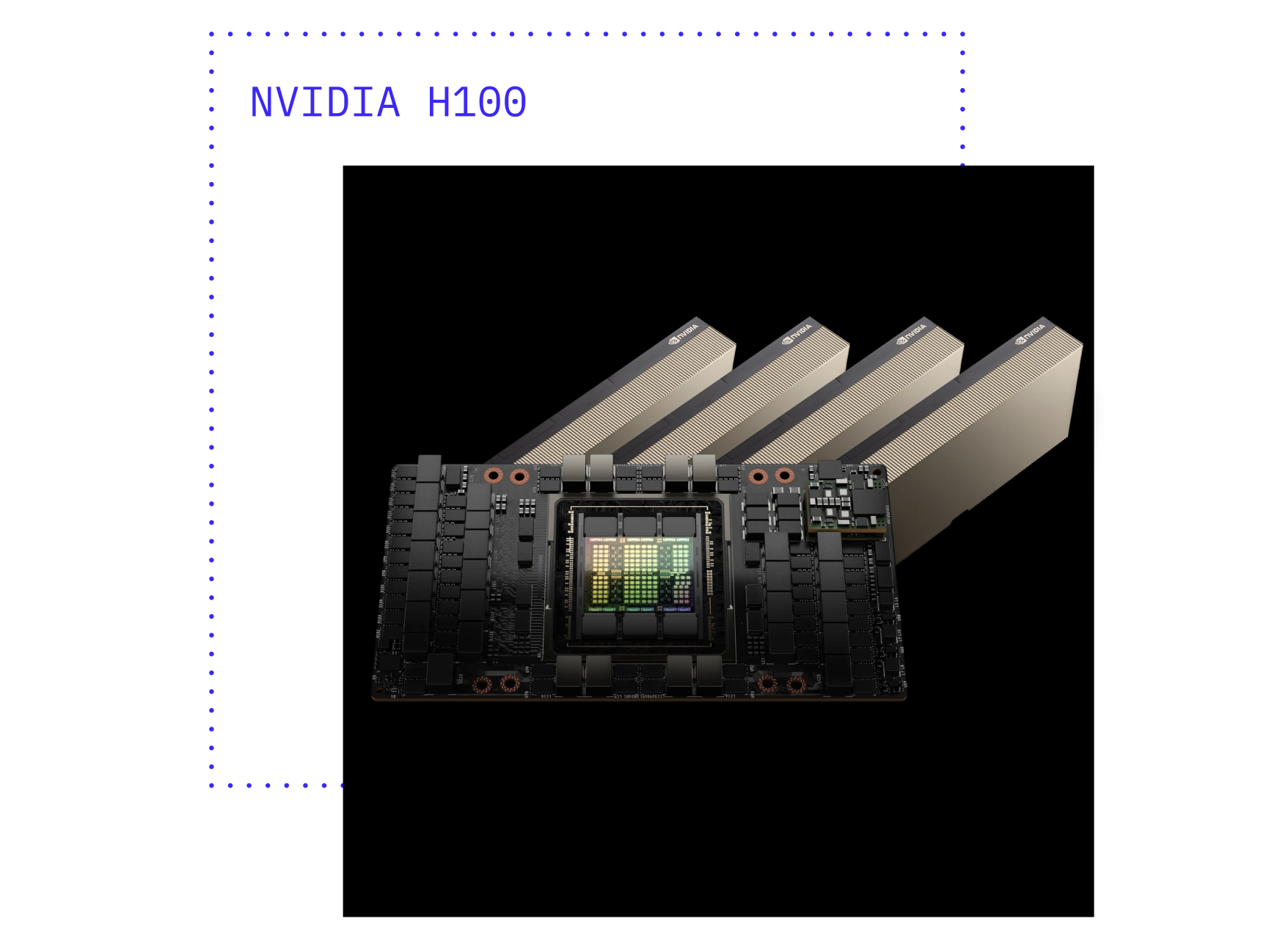
Ready to get started?
Create a cloud account instantly to spin up GPUs today or contact us to secure a long-term contract for thousands of GPUs